Benford's Law: An empirical finding & its applications in risk
The frequencies of the first digits of numbers related to various “real-life” phenomena — social media follower counts, river lengths, and financial amounts — follow a specific pattern known as Benford’s Law.
This pattern was named after the scientist F. Benford, who published a comprehensive paper on it in 1938. According to Benford’s Law, digit “1” appears as the first digit 30.1% of the time, followed by digit “2” at 17.6%, and so on, with digit “9” appearing just 4.6% of the time.
This finding dates back to 1881, however, as it was another scientist, S. Newcomb, who first noticed that the pages of logarithm tables (written records of logarithm values from the pre-computer era, very used in fields like astronomy or navigation) were not worn equally: the page referring to lower digits appeared as much more used than other ones.
Benford's Law is a valuable tool for banks, fintechs, and lenders because it can immediately flag suspicious frequencies. If a set of financial transactions deviates sensibly from the expected pattern, it could mean that there is something unusual.
Throughout history, Benford’s Law has been widely used to test the legitimacy of financial statements in cases of large datasets, including governmental macroeconomic data submitted to the EU, corporate financial records, and the predictability (or lack thereof) of financial markets.
How we use Benford’s Law at Inscribe
At Inscribe, we apply Benford's Law to analyze the frequency of amounts on bank statements, combining it with AI and statistics derived from our own data to identify any unusual digit patterns. When deviations from expected frequencies occur, it’s crucial to determine whether these are natural variations due to specific data characteristics or if they suggest something suspicious. In other words, we need to quantify how much deviation is too much.
In the documents that Inscribe has deemed as non-fraudulent, Benford’s Law generally holds true with only slight deviations. For example, the relatively high frequency of the digit “5” might be due to small bank charges that naturally occur more/less often in our data.

The plots below illustrate different situations: a document with good Benford’s Law alignment and one where some of the digits display a peculiar behavior far from expectations.
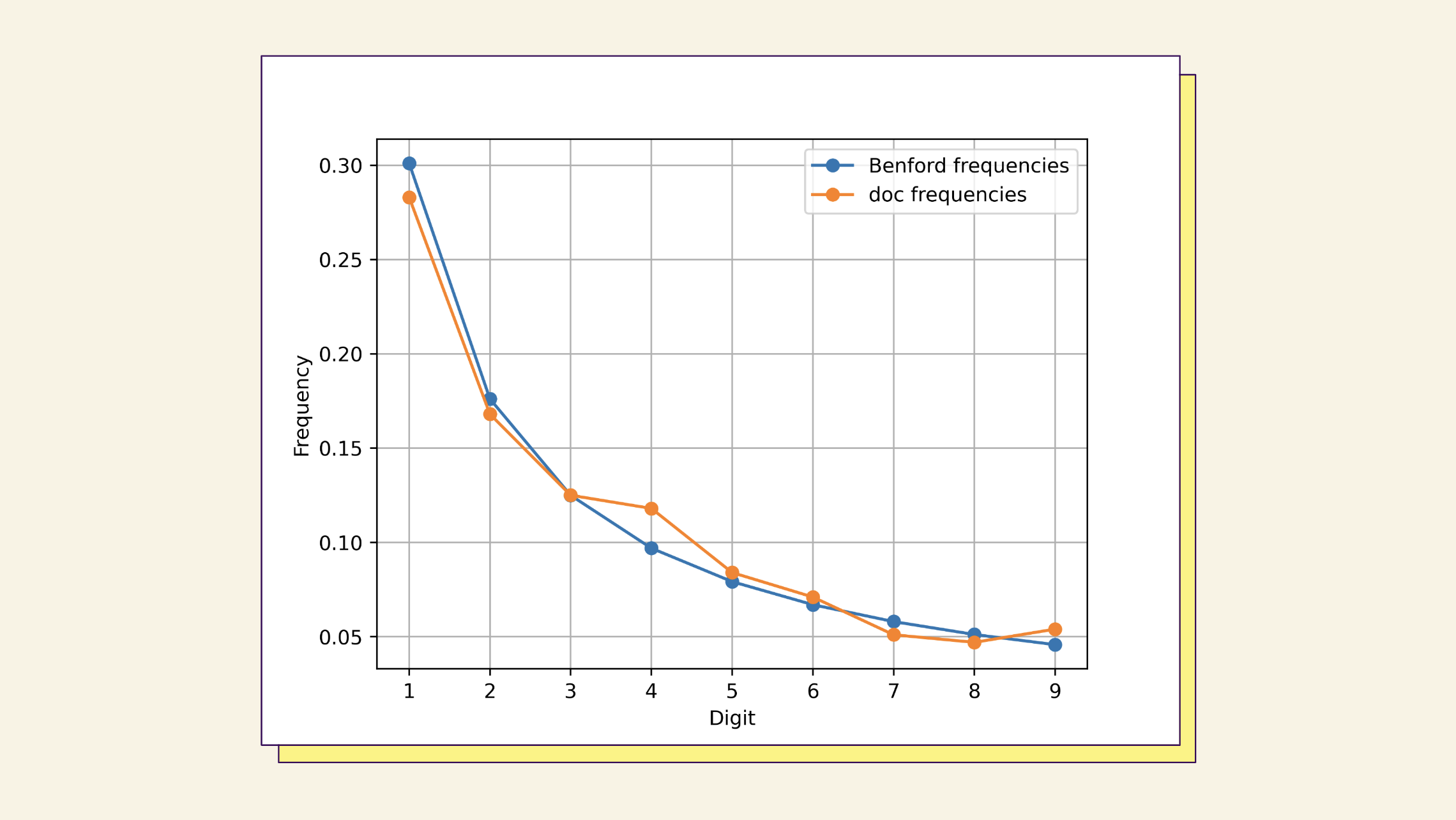

To determine if a document violates Benford's Law, we use information from non-fraudulent documents to calculate the distributions of deviations from Benford’s Law frequencies for each digit. On a new document, we compute said deviations and check how they fare in regard to these distributions. Deviations that are not in line with the ones from non-fraudulent documents are unusual.
Of course, we can only run Benford’s Law check on documents where the digit frequencies can be computed in the first place because the documents have enough transactions. Again, we use our data and statistics to derive a threshold.
The role of Benford’s Law in our AI Fraud Analyst’s risk report
Our AI Fraud Analyst uses Benford’s Law within its analysis of transaction amounts. Like the other verifications we run on documents, our AI Fraud Analyst compiles the results in an easy-to-digest and auditable summary. If certain digits deviate from Benford’s expected pattern, the summary will highlight this:

It is important to understand that a single document not respecting Benford’s Law is not necessarily an indication of fraud. There can be legitimate reasons why a statement has first-digit frequencies that are far from expectations. For example, someone may have purchased the same thing repeatedly for a period of time, they may have several bank charges for the same amount, or they may regularly receive similar-amount payments in small chunks, one at a time.
Because of this, the Benford’s Law check is typically a low risk by itself. When something suspicious is detected, it will provide fraud analysts with additional insights to better inform their own decision-making. On the other hand, in cases where it does not find anything suspicious, it naturally helps to categorize the document and the applicant as low risk.
Want to learn more about our AI Fraud Analyst? Reach out to book a demo and speak with an AI expert from our team.
About the author
Martina works as Senior Research Scientist at Inscribe, where she investigates the use of state-of-the-art techniques in Statistics and AI to fraud detection. She holds a PhD in Physics and is very passionate about data and creating automation to solve real-life problems.
Deploy an AI Risk Agent today
Book a demo to see how Inscribe can help you unlock superhuman performance with AI Risk Agents and Risk Models.