Article
Credit Analysis: A Complete Guide
In this post, we take a closer look at traditional credit analysis and explore how technology is playing a role in reshaping this critical capability.
- Loading table of contents...
Credit analysis – the process of evaluating the creditworthiness of a borrower and the likelihood that they will repay their debts – plays a pivotal role in helping banks, financial institutions, credit agencies and lenders of all kinds make informed decisions about extending credit, managing risk, and ensuring the stability of financial markets.
Unfortunately, credit risk isn't always easy to determine, especially when relying on traditional, manual processes. This is because borrower applications often contain missing, complex or even fraudulent documentation, thus making it impossible for analysts to conduct a thorough evaluation.
However, advances in data analytics and technology are helping organizations automate key parts of the credit analysis process, which not only saves them time but also helps reduce risk and improve decision-making.
In this post, we take a closer look at traditional credit analysis and explore how technology is playing a role in reshaping this critical capability.
What Is Credit Analysis?
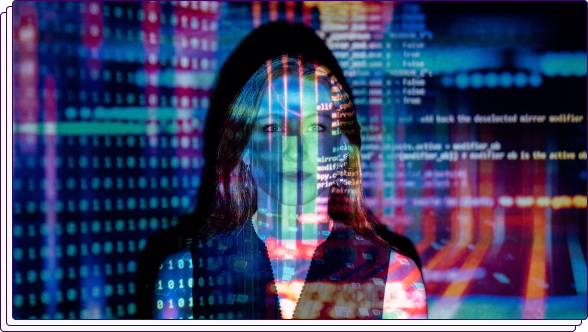
Credit analysis is a structured and systematic approach to evaluating the risks associated with lending. The process varies depending on the type of borrower involved—consumer, corporate, or sovereign – and focuses on different factors and considerations due to the unique characteristics of these borrowers.
- Consumer credit analysis evaluates the creditworthiness of individuals who are applying for loans, such as mortgages, car loans, and credit cards. Factors include the borrower's income, expenses, debt obligations, credit history, and employment history.
- Corporate credit analysis evaluates the creditworthiness of businesses that are applying for loans, such as lines of credit, term loans, and bonds. Factors include the company's financial statements, industry outlook, management team, and competitive landscape.
- Sovereign credit analysis evaluates the creditworthiness of countries that are issuing debt, such as government bonds. Factors include the country's economic outlook, political stability, and debt burden.
Credit Analysis Process
While the steps involved in credit analysis can vary, they typically include the following key areas:
- Gathering relevant information: The first step in any credit analysis process is to gather information such as financial statements, credit history, income, and expenses to establish a credit profile.
- Performing a thorough analysis: This involves evaluating a variety of factors that are specific to the applicant, such as their financial health, as well as macroeconomic factors, such as the industry outlook and competitive landscape.
- Developing a credit rating: The credit analyst will then develop a credit rating based on their analysis that is used to determine the interest rate.
- Making a lending or investment decision: The analyst then makes a determination based on the credit rating relative to the lender’s risk tolerance and investment objectives.
The 4 Cs of Credit Analysis
The "4 Cs of Credit" is a widely used framework in credit analysis that helps assess the creditworthiness of borrowers. These four key factors provide a structured way to evaluate the risk associated with lending money or extending credit.
The 4Cs of credit analysis are:
- Character: Refers to the borrower's previous financial track record and their willingness and ability to repay the debt.
- Capacity: Refers to the borrower’s ability to generate cash flow to repay the debt.
- Capital: Refers to financial resources and the amount of money they have invested in the transaction, such as a down payment.
- Collateral: Refers to assets that can be leveraged in the event of default.
Tools & Techniques
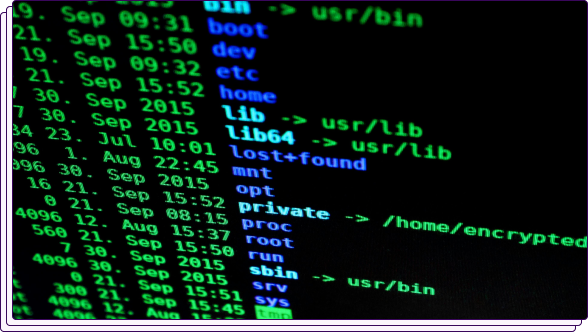
There are a variety of tools and techniques that can be used to conduct sound credit analysis. Some of the most common tools include:
- Financial ratios: Analyzing financial statements to assess financial health. This includes looking at factors such as income, expenses, assets, and liabilities.
- Cash flow analysis: Assessing the borrower’s ability to generate cash flow to repay the loan. This includes looking at factors such as revenue, expenses, and working capital.
- Debt-to-equity ratio: A measure of a borrower's leverage and includes factors such as amount of debt owed, the interest rates being paid, and the maturity dates of their debts. A higher debt-to-equity ratio indicates that the borrower is more leveraged and therefore could be riskier.
- Credit scoring: A statistical method for assessing a borrower's creditworthiness. Credit scores are based on a variety of factors, such as financial statements, credit history, and industry outlook.
The techniques that are used during the credit analysis will vary depending on the type of lender, borrower segment, and the type and amount of credit being requested. For example, a lender might use financial statement analysis and credit scoring to evaluate a small business loan, while an investor might use debt analysis and industry analysis to evaluate a bond investment.
Key Metrics & Ratios

As mentioned above, ratios are an essential component of credit analysis. Key ratios used during the credit analysis process include:
- Debt-to-equity ratio
- Current ratio
- Debt service coverage ratio
- Earnings before interest and taxes (EBIT)
- Debt/EBIT Ratio
Debt-to-equity ratio measures the amount of debt a company has relative to its equity. A higher debt-to-equity ratio indicates that the company is more leveraged, which could mean it has a higher risk of default.
Current ratio measures a company's ability to pay its short-term debts with its current assets. A higher current ratio indicates that the company is more liquid, which could mean it has a lower risk of default.
Debt service coverage ratio measures a company's ability to cover its debt payments with its operating cash flow. A higher debt service coverage ratio might indicate a company is more likely to be able to repay its debts, which could mean it has a lower risk of default.
Earnings before interest and taxes (EBIT) measures a company's operating profit. A higher EBIT could indicate that the company is more profitable and might have a lower risk of default.
Debt/EBIT ratio measures the amount of debt a company has relative to its EBIT. A higher debt/EBIT ratio could indicate the company is more leveraged, which could mean a higher risk of default.
Credit Rating Agencies
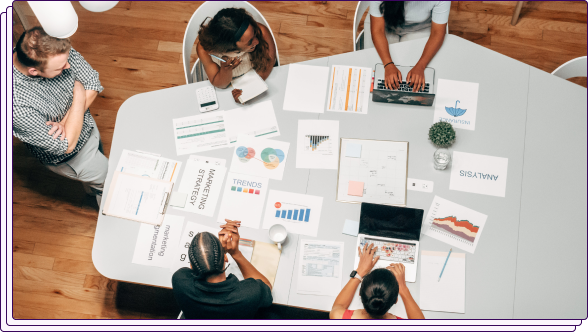
Credit rating agencies are another player in the credit analysis space. They assess creditworthiness by assigning credit ratings to entities, which are then used to assess lending risks.
There are three major credit agencies: Moody's, S&P Global Ratings, and Fitch Ratings. Known as the "Big Three,” these organizations assign their ratings using a scale that ranges from AAA (highest credit quality) to D (default). The higher the credit rating, the lower the risk of default. It's important to note that while these agencies provide valuable insights into credit risk, they are not infallible, and their ratings should be used as part of a broader due diligence process.
A recent example of the far-reaching impact of a credit rating action was the downgrading of the long-term credit rating of the United States from AAA to AA+ by Fitch Ratings. Fitch said that the downgrade reflected "the increasing uncertainty around the government's ability to manage its fiscal challenges.” The downgrade had a significant impact on financial markets and created a ripple effect around the world: the stock market fell sharply; the interest rate on U.S. Treasury bonds rose; and concerns about the global economy increased.
Credit Analysis by Industry
Credit analysis varies by industry. This is because the factors that affect creditworthiness can vary depending on each sector.
For example, factors that are important for assessing the creditworthiness of a bank will be different from factors used to assess those of a real estate developer or an energy company. Banks are typically evaluated based on their capital strength, liquidity, and asset quality, whereas real estate developers could be assessed on financial strength, experience, and track record. Energy companies might be rated on their reserves, production, and financial strength.
The credit analyst will need to consider all relevant factors when conducting a credit analysis to make informed decisions that minimize the risk of loss.
Challenges and Risks
Credit analysts face a number of challenges and risks in their work. Key issues include:
- Incomplete or inaccurate applications: Credit analysts routinely work with incomplete or inaccurate data – sometimes without knowing this is the case. This can make it difficult to assess the creditworthiness of borrowers.
- Complexity of financial statements and products: The emergence of increasingly complex financial products and instruments, such as derivatives, structured products, and various types of securities, makes it more challenging for analysts to review and understand financial statements, as well as identify and calculate potential risks.
- Fraud: An uptick in document fraud – when individuals or businesses submit fake or manipulated statements as part of their application – has made it difficult for analysts to accurately evaluate the borrower.
- Time constraints: Credit analysts often need to make decisions quickly, which limits the amount of time they have to review and analyze documents or conduct other steps within the analysis process.
- Bias: Credit analysts can be biased in their assessments, which can lead to inaccurate ratings or assessments.
Strategies for Mitigating Risk
Despite these challenges, there are proven strategies lenders can consider to help mitigate risk and improve decision-making:
- Diversification: One way for organizations to effectively lower risk is by diversifying their portfolio across different types of borrowers, industries, and asset classes. This effectively spreads the organization’s credit risk and helps to ensure the agency will not be impacted by a singular event or factor specific to any one customer segment.
- Scenario analysis: As part of the evaluation process, organizations should consider various economic scenarios, including downturns and adverse market conditions, that may impact the credit risk of the borrower.
- Data analytics, modeling and intelligent automation: Companies should not rely exclusively on human analysts to evaluate and assess risk. Advanced data analytics, predictive modeling and intelligent automation tools, including AI, can help companies automate the analysis of large datasets to identify patterns and trends that often cannot be identified by humans. This helps to improve both the speed at which the credit analysis is conducted, as well as the accuracy of the evaluation.
- Document fraud detection tools: Credit agencies should also conduct extensive due diligence and verify the accuracy of all information provided during the application process. This includes verifying the authenticity of any documentation submitted by the applicant, such as bank statements, paystubs, investment portfolio statements, tax forms, or even identification documents. Most signs of document manipulation are impossible to detect with the human eye. It is extremely important that companies leverage advanced technology to enable automated document reviews, which can quickly scan materials provided by the applicant and verify their authenticity.
Emerging Trends: Future of Credit Analysis
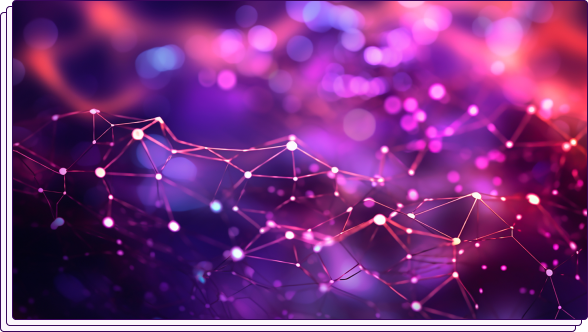
Credit analysis is a complex task made even more complicated by the evolving nature of the landscape. Looking to the future of credit analysis, recent developments and technological advancements are reshaping the industry and making it easier for credit analysts to assess creditworthiness and mitigate risks quickly and more accurately.
- Artificial intelligence (AI) and machine learning (ML) are being used to automate tasks, improve data analysis, mitigate risk and decrease time needed for review. For example, AI can be used to analyze large amounts of data to identify patterns and trends that would be difficult to identify manually. ML can be used to develop models that can predict the likelihood of default.
- Cloud computing and big data is being used to gather, process, and store large amounts of data, as well as produce insights that analysts can act on.
- Blockchain is being used to create a secure and transparent record of financial transactions. This can be used to improve the efficiency and accuracy of credit analysis.
- Natural language processing (NLP) is being used to analyze text data, such as social media posts and news articles. This can be used to assess borrowers' financial health and risk of default.
- Robotic process automation (RPA) is being used to automate tasks, such as data entry and document processing. This frees up credit analysts to focus on more complex tasks, such as analysis and decision-making.
A Closer Look at the Impact of AI and ML on Credit Analysis
AI/ML are having a major impact on the credit analysis field. These technologies are being used to automate tasks, improve data analysis, and identify potential risks while also reducing the time it takes to make a credit decision.
Some specific examples of how AI and ML are being used in credit analysis include:
- Credit scoring: AI and ML are being used to develop credit scoring models that can predict the likelihood of default. These models are more accurate than traditional credit scoring models and can help lenders to make better lending decisions.
- Fraud detection: AI and ML are being used to detect fraud in credit applications, such as through the use of document fraud detection tools. This can help lenders protect themselves from losses due to fraud.
- Risk management: AI and ML are being used to manage risk in credit portfolios. This can help lenders to identify and mitigate risks, such as the likelihood of default.
- Compliance: AI and ML are being used to automate compliance activity. This can help lenders avoid penalties for non-compliance.
Final Thoughts on Credit Analysis
Credit analysis is a critical tool for lenders and investors to make informed decisions that can minimize loss and maximize returns. While not a perfect science, credit analysis is an essential tool for mitigating risk and protecting financial assets. Tools like artificial intelligence and machine learning have the potential to revolutionize the field by helping credit analysts make better decisions faster.
Inscribe is committed to helping financial institutions, credit agencies and lenders of all types improve the speed and accuracy of the credit analysis process through automated document reviews for files like bank statements, credit card statements, pay stubs, tax documents and driver's licenses.
Inscribe’s automated fraud analysis enables your team to make quicker and more precise decisions in a matter of seconds by instantaneously detecting issues within fake and manipulated documents that are often invisible to the human eye.
To learn more about how Inscribe can help your company automate time-consuming and complex document fraud reviews, improve the speed and accuracy of reviews and reduce loan write-offs, contact us to schedule a personalized demo today. It’s the one step in the credit analysis process you can’t afford to miss!
Curious to see how it all works? Speak with one of our experts and set up a personalized demo today.