This receipt was made by AI — can you tell?
The technology behind AI-generated images has taken a leap forward.
This receipt was made using a generative image model via a simple prompt. Can you tell? While visually compelling at first glance (realistic lighting, paper texture, crinkled appearance) closer inspection reveals several red flags.
- The receipt lists a MacBook Pro purchase from an “Apple Store Grafton Street,” which does not exist (Apple has no official store at that location).
- It also contains minor typographical errors such as “VSA” instead of “VISA” as the payment method, and inconsistent number formatting (e.g., U.S.-style comma/period usage in Euro-denominated amounts).
- The subtotal jumps unexpectedly by $2,000, despite the tax and total amounts being correctly calculated.

Recent updates from OpenAI have made it easier than ever to create these photorealistic receipts, invoices, and other financial documents (many of which could be used for fraudulent purposes).
The big technical leap here comes from new autoregressive models that generate images pixel by pixel (instead of refining noise like diffusion models). This method enables a different class of visual realism; one that’s eerily convincing, especially at first glance. And while this is impressive from a research perspective, it introduces new risks for any business that relies on document-based workflows.
Whereas a few years ago, image generation was more novelty than threat, it’s now clear that generative models are on a path toward creating documents that could easily fool a human reviewer. It’s not a matter of if this will happen, but when.
At Inscribe, we’ve been tracking this shift closely. We’ve tested these tools, reverse-engineered the outputs, and developed new detection systems built specifically for this next generation of fraud.
Here’s what we’re seeing, and how we’re responding.
Are AI-Generated documents a real threat today?
The short answer: not quite yet. But we’re getting close.
Today’s models are still prone to making small but significant mistakes — like typos in names or addresses, currency formatting errors, or inconsistencies between metadata and visual content. We’ve seen invoices where the math doesn’t add up and receipts with non-existent store locations.
These mistakes are usually enough for manual reviewers (or advanced fraud detectors) to flag them. But this won’t last forever. The pace of improvement in AI models means these errors are being ironed out quickly. And even if a model can’t generate an entire fake document convincingly, fraudsters can still use it to synthesize pieces — like fake transaction histories or modified logos — and then manually blend them into real documents.
What we’re dealing with is a highly dynamic threat that requires constant vigilance and innovation. And that’s exactly what we’re focused on at Inscribe.
Our Approach: Detecting the Undetectable
Our mission has always been to enable instant trust in financial services. That means not only detecting traditional fraud, but staying ahead of entirely new forms of deception, like AI-generated documents.
Thankfully, the rise of generative AI hasn’t left us powerless. It’s opened new doors. These synthetic documents contain unique fingerprints: pixel-level artifacts, unnatural lighting, suspicious shadows, text inconsistencies, and more. With the right tools, these signatures are detectable.
We recently released our "AI Generated" fraud detector, which flags documents likely generated by AI using digital signatures and pattern recognition.

And we're continuously expanding our multi-layered approach to AI fraud detection:
- Forensic analysis: Identifying visual artifacts, typographic patterns, and layering inconsistencies
- Metadata validation: Comparing embedded document data with content and context
- Semantic modeling: Using LLMs to detect logical inconsistencies or anomalies in the content
- Network detection: Identifying document patterns across our global network to flag emerging fraud trends
- Synthetic dataset training: Generating thousands of AI-created fakes in-house to train and improve our detectors
Our research & data science team is actively researching how these models behave, what patterns they leave behind, and how we can train our systems to stay one step ahead, even when those systems include other LLMs.
Most common AI-generated document types
Our research shows that utility bills, invoices, and bank statements make up 70% of AI-generated document fraud attempts.
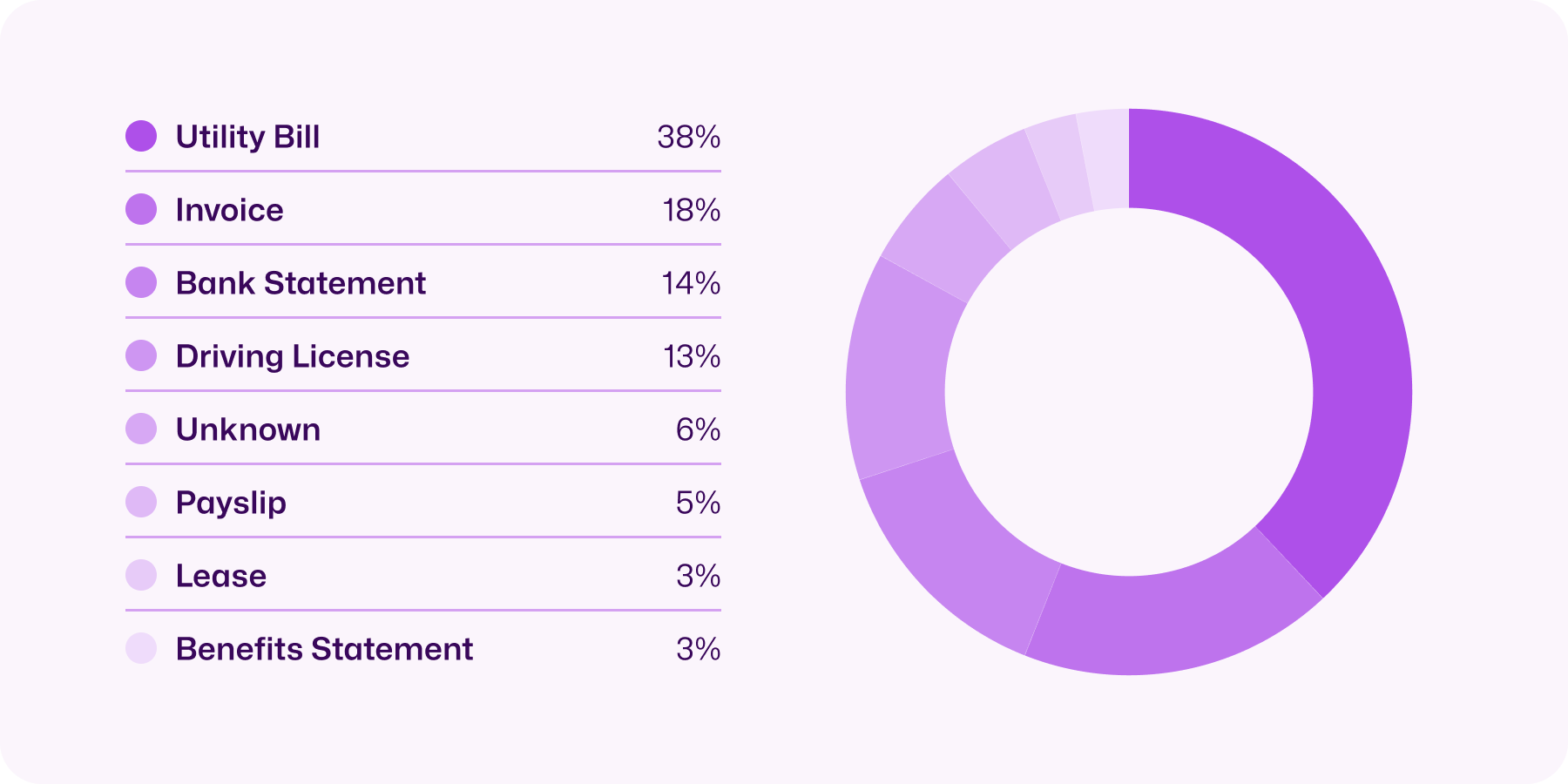
Document Trust in the Age of AI
This moment isn’t just about new threats. It’s about how we evolve our systems to meet the future of trust.
Historically, we’ve relied on visual inspection and metadata to validate documents. But as AI-generated content becomes more sophisticated, that won’t be enough.
In the near future, we may need cryptographic proof of authenticity: a digital chain of custody that links documents back to their source of origin (like a payroll provider or bank). We’re not there yet, but it’s clear that visual resemblance is no longer a sufficient marker of truth.
This future raises an important shift: Instead of asking “Does this document look fake?”, we’ll need to ask “Can this document prove it’s real?”
Looking ahead, I’m confident that AI-generated documents will become more common and more convincing. But I’m also confident that they will remain detectable, and that with the right systems in place, they can be stopped before they cause harm.
The future of financial services will be built on a foundation of real-time risk analysis, AI-augmented decision making, and trusted digital systems. At Inscribe, we’re building that future every day; not just by reacting to change, but by anticipating it.
To the fraud fighters, risk leaders, and AI builders reading this: stay vigilant, stay curious, and stay ahead.
We're just getting started.
If you’re interested in learning more, we’d love to have a conversation. Simply reach out to schedule a meeting with our team.