Article
Anti Fraud Software: How AI Can Help
Advanced detection methods made possible anti-fraud AI helps financial institutions combat today’s sophisticated criminals and reduce fraud losses
- Loading table of contents...
In the latest edition of their annual Global Economic Crime and Fraud Survey, PricewaterhouseCoopers (PwC) estimates that nearly half of companies have experienced fraud, with an average of six instances per company.
The report estimates the total cost of fraud, including customer fraud, cybercrime, and asset misappropriation, to be a staggering USD $42 billion. In this article, we explore the latest in fraud detection capabilities and how companies can benefit from AI anti fraud software.
What is fraud detection?
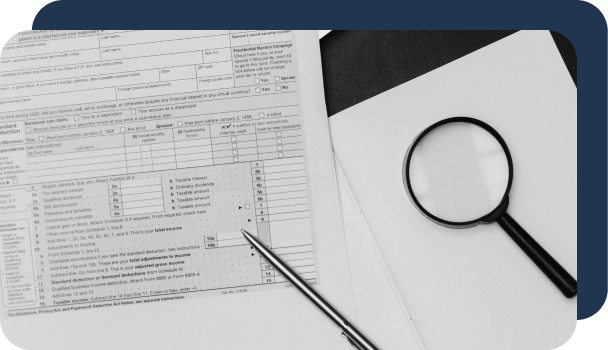
Fraud detection is the collective term for any processes, procedures, tools, or systems that help businesses and other organizations identify or prevent fraudulent or unauthorized activity.
Examples of fraud
Fraud can take many forms and affects virtually every industry. In most cases, the goal of such schemes is to obtain money or property under false pretenses. Common examples include:
Fraud detection methods
Many criminals who commit fraud do so by following the same formula for each scheme. As such, it is possible to recognize the pattern of fraudulent activity and stop it before any money or property is lost.
Advances in digital technology have greatly enhanced fraud detection capabilities. Modern solutions, which leverage algorithms as the foundation for predictive analytics and machine learning models, can review millions of transactions, events, and other data points in real-time to identify patterns that can indicate fraudulent activity. Since most machine learning models are capable of “learning” over time, the tools become more accurate and precise in their detection capabilities.
At that same time, the increasingly complex and global nature of our lives, as driven by the advent of the internet and other digital technologies, has made it easier for criminals to disguise their activity. For individuals, increased travel, online shopping, and use of digital banking and other e-services can provide new inroads for adept cybercriminals. For companies, the rise in remote work, as well as other significant organizational changes, such as mergers, acquisitions, the shift to the cloud, or new system integrations, can create vulnerabilities and weaken the business’s ability to detect fraud.
n this landscape, it is essential for organizations to embrace anti fraud AI-powered solutions to anchor their fraud detection practice.
Anti-fraud data analysis techniques

Data analysis is at the heart of every modern fraud detection tool. Data-based anti-fraud capabilities can generally be categorized into two groups:
- Statistical data analysis techniques
- Artificial intelligence (AI) analysis techniques
Anti-fraud statistical analysis techniques
“Statistical analysis techniques” refers to any tool that leverages data analytics to identify patterns based on past data to predict future events. These methods include:
- Statistical parameter calculation: Using data to calculate a wide range of statistical parameters, such as averages, quantiles, distributions, probabilities, and other metrics, that can be used to pinpoint potential instances of fraud within a data set.
- Regression analysis: A comparative technique that explores the relationship between two or more dependent or independent variables to identify behavior patterns and predict potentially fraudulent activity.
- Probability distributions and models: A mapping technique that visualizes all possible values and likelihoods or a variable within a given range.
- Data matching: A technique that compares two data sets to identify links for a variety of purposes, including fraud detection.
Anti-fraud AI analysis techniques
Advances in AI technologies has made anti-fraud AI solutions an essential part of every organization’s fraud detection strategy. Unlike statistical analysis tools, which compare data to existing models, AI-enabled analysis tools are capable of making assumptions or even using complex reasoning to build their own models.
AI-enabled fraud detection techniques include:
- Pattern recognition: Leverages ML-based algorithms to automate the recognition of patterns and regularities in data.
- Data mining: Combines statistical analysis tools with AI algorithms to review a large data set, including sorting and segmenting data points, to identify patterns.
- ML fraud detection: Algorithm-enabled tooling that leverages historical data to identify patterns that can be detected in the future.
- Neural networks: A tool modeled after the human brain that enables critical reasoning that enables computer programs to recognize patterns and detect specific instances in the future. Unlike ML models, which can only take action based on what the model is “taught”, neural networks are capable of learning and making logic-based decisions on their own.
Benefits of AI for Fraud Prevention

Leveraging anti-fraud AI generally offers organizations two main benefits:
- Improved detection time
- Enhanced detection accuracy
Manual fraud detection systems, which relied largely on human analysts, are no longer a practical or effective solution for keeping up with the explosion of digital transactions occurring in the modern landscape – every one of which can be subject to fraud.
Anti-fraud AI tools and techniques offer organizations real-time data analysis, enabling them to block potentially fraudulent activity or require verification before proceeding. As models take in more and more data, their analysis capabilities become more precise, which helps them become more accurate over time and reduces the number of false positives within the system.
In addition to improving detection time and accuracy, anti fraud AI and ML-enabled tools also help automate other tasks that were once managed manually. These include:
- Formulating and updating effective rules and alerts
- Automating key aspects of the investigation process, such as data collection and querying
- Prioritizing activity and optimizing resources based on the severity of the issue
- Fast-tracking or resolving routine or low-risk cases through automation
- Improving the customer experience with a faster, personalized service
- Visualizing data through knowledge graphs or other tools
- Autofilling any necessary paperwork or forms needed during the investigation or case resolution process
Use AI to fight fraudulent activities with Inscribe
According to the PwC economic crime and fraud study cited earlier, just over half (56%) of organizations that were the victim of fraud or other e-crime conducted an investigation into their worst incident. This point, coupled with the fact that nearly half of companies experienced at least one confirmed case of fraud, underscores the idea that companies need to do more to ramp up their fraud detection capabilities and invest in solutions that will help prevent such instances in the future.
Many cases of fraud are entirely preventable with the right tools and technologies. At Inscribe, we're building fraud detection tools to enable high-trust online use cases. We work with some of the world's fastest-growing fintechs and numerous Fortune 500 companies, helping them to reduce fraud and give quicker decisions to their customers.
Want to learn more about how Inscribe can help your organization combat fraud? Contact our sales team to schedule a personalized demo.